< BACK TO ALL BLOGS
NEXTDATA Solutions:80% direct reduction, how can the image-text sentiment analysis model save the cost of human review?
Aug 2, 2023
Case 1: On a dating social software, the guest’s profile page displayed “work in the municipal
government” with a “flag sticker” in the lower right corner of the avatar. When chatting with the match partner, it revealed that “I work in a government agency…”
Case 2: During the Winter Olympics, Chinese athletes won the gold medal and wore the national flag, which aroused heated discussions. Netizens on a certain social platform were also infected and actively created pictures to express their patriotic feelings. Among them, a small number of pictures of damaged national flags were also mixed in...
This is a common scenario in the audio and video social industry, where user profiles, published content, and private message conversations may all cause risks. Usually, the platform adopts a high recall strategy and conducts manual review. The advantage of doing this is that the accuracy rate is high, but from the perspective of user experience and labor cost, this is not a good choice, especially when a large amount of content is generated, the manpower may be overloaded, and audit errors and omissions may occur.
If sentiment analysis is performed on text and pictures, after the algorithm screens the question pictures and texts, and then conducts manual review, the labor cost may be greatly reduced.
Case 3: In a beauty brand mall, a user’s evaluation of a popular product is: “Moisturizing water is very moisturizing, suitable for winter use, but too greasy for summer use”This is a common scenario in the e-commerce industry. Sentiment analysis on product reviews can help brands quickly understand the
direction of public opinion and adjust their response strategies.
Sentiment analysis is usually applied to film reviews, e-commerce evaluations, and public opinion analysis. With the continuous advancement of technology, the requirements for machine review of sensitive content in the market continue to increase. The text sentiment positive and negative analysis model recently launched by NEXTDATA has helped customers reduce manpower review costs by 80%, and the picture sentiment positive and negative model, which gives priority to negative pictures, has helped customers reduce manpower review costs by 90%.
This article will introduce the necessity of sentiment analysis, the method and application of NEXTDATA for text and picture sentiment analysis.
1. Why Do Sentiment Analysis?
Supervision, public opinion monitoring, platform UGC content analysis, all aspects have put forward higher requirements for the review and analysis of graphic content.
It is difficult to identify the positive and negative emotions in the content only by the "blacklist", and it is also easy to "manslaughter" non-negative information, such as "I work in a government agency..." mentioned above, which affects user experience.

In addition, during major events, such as floods, epidemics, etc., the emotions and opinions carried by social networks will have an increasing impact on society, mixed with true and false information, and netizens’ emotions are intense. The sentiment analysis of text/pictures is of great significance, whether it is public opinion in progress or after the event. Review guidance [1]. In addition, a large number of speeches and pictures produced intensively at critical times will put great pressure on human review, and the "keyword" mechanism may further trigger a crisis of public opinion, requiring the coordinated operation of machine review + human review, and even adding the proportion of
manual content recommendation by manual editing, such as the exploration of Meta (Facebook), Twitter and Facebook in recent years.
From the perspective of business scenarios, e-commerce and local life platforms that involve product and service evaluation also need sentiment analysis and guidance based on the analysis results. For example, a mobile phone brand's self-operated shopping mall, a certain car brand's own community, etc.,
will have evaluations for "X brand X performance". In complex cases, there will be multiple evaluation objects, such as multiple performance characteristics of X brand, or comparison with other brands. Positive and negative sentiment analysis helps brands monitor the direction of public opinion, adjust decisions, and improve products.
2. Human Review Costs for Text Sentiment Analysis are Reduced by 80%
Text sentiment analysis refers to the process of analyzing, processing and extracting subjective text with emotional color by using natural language processing and text mining technology.
It contains five elements: entity , aspect, opinion , holder , and time . What needs to be answered is a
question: "Who? When? For what? Which attribute? What kind of emotion is expressed?" The influence of the expresser and the time of expression basically does not affect the final evaluation attitude, so sentiment analysis mainly focuses on entities, attributes, and opinions.
Example sentence: The intelligent control system of XX car is very advanced.
XX Car: Entities
Intelligent Control System: Properties
Advanced:Perspectives
Text sentiment analysis tasks include word-level sentiment analysis, sentence-level sentiment analysis, and target-level sentiment analysis. The word-level and sentence-level analysis is the positive and negative sentiment of a word or the entire sentence, and the specific entities and attributes in the text are not separately distinguished. If the text is more complex, or requires higher accuracy, it is necessary to make a judgment on the specific evaluation object in the text, that is, target-level text sentiment analysis. At present,
NEXTDATA has been able to realize the positive and negative judgments of the six targets (evaluation objects) in the text.
Example:
Word level:birthday - positive
Sentence level:XX course has a lot of dry goods and detailed content——Positive
Target class:
1. The instrument panel of the XX series is not good-looking at all. The car as a whole looks good-the instrument panel (attribute)-negative; the whole car (attribute)-positive
2. I support the Chinese government’s accusation of country X’s policy—Chinese government (attribute) – positive; country X’s policy (attribute) – negative
For example, in the dating community, scenarios such as nicknames, personal introductions, and private chats are common risk scenarios. After a customer accesses
the positive and negative sentiment analysis model of NEXTDATA, the cost of human review is greatly reduced by 80%. In an online community, the novel text is the core risk scenario. In the past, keywords were used to filter the text. After a customer connected to the positive and negative sentiment analysis model, the accuracy rate increased by 30 times in the same scenario.
3. Image Sentiment Analysis Reduces Human Review Costs by 90%
The sentiment analysis of pictures is based on the massive picture data of NEXTDATA , and the picture classification model is customized through deep learning methods. For specific occasions, classification labels are added to classify and identify negative content. The ability to process hundreds of millions of pictures per day makes model iteration faster and more efficient. For example, the national flag is a "sensitive" content. Through the "national flag + violence" label, the model can identify negative images that damage the national flag.
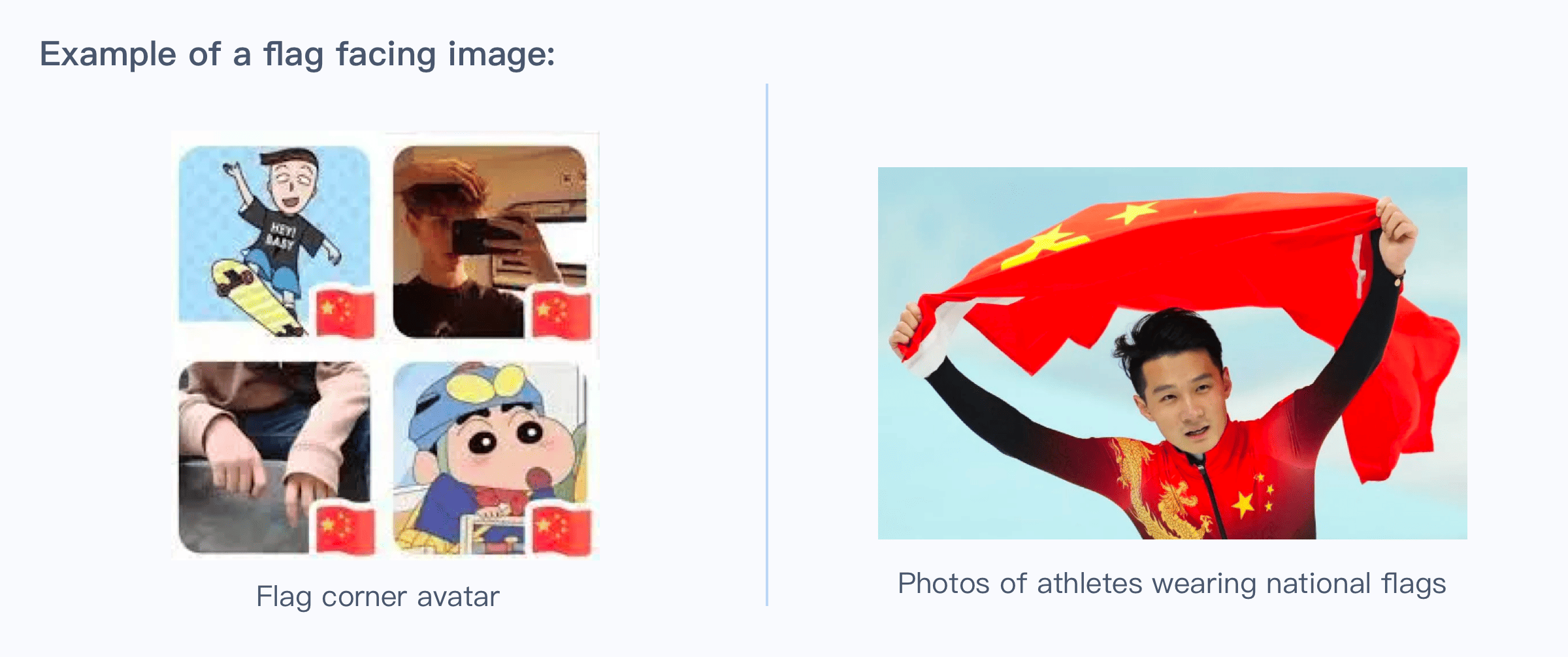
For example, a platform generates 20,000 national flag-related pictures every day. During large-scale events, such as the recent Winter Olympics, the content related to the national flag may show a blowout state, and a small amount of pictures of damaged national flags may be mixed in the front of athletes wearing the national flag. The pressure of human review is high, and the probability of errors and omissions is high. At this time, the positive and negative model is introduced, and the "machine review + human review" mechanism is used to implement the first layer of filtering of negative content, identify 400 of them that may be risky, and give priority to human review of negative content to improve review efficiency.
Taking a document tool as an example, user avatars and documents are mass-produced and edited by users. Originally, 100,000+ national flag-related pictures were generated in a single day, all of which needed to be done manually. After accessing the positive and negative sentiment analysis of NEXTDATA pictures, a single The daily review volume of negative images related to the national flag was reduced to 400, and manual review of negative content was prioritized, reducing the cost of human review by more than 90%.